Understanding Machine Learning Annotation and Its Impact on Business
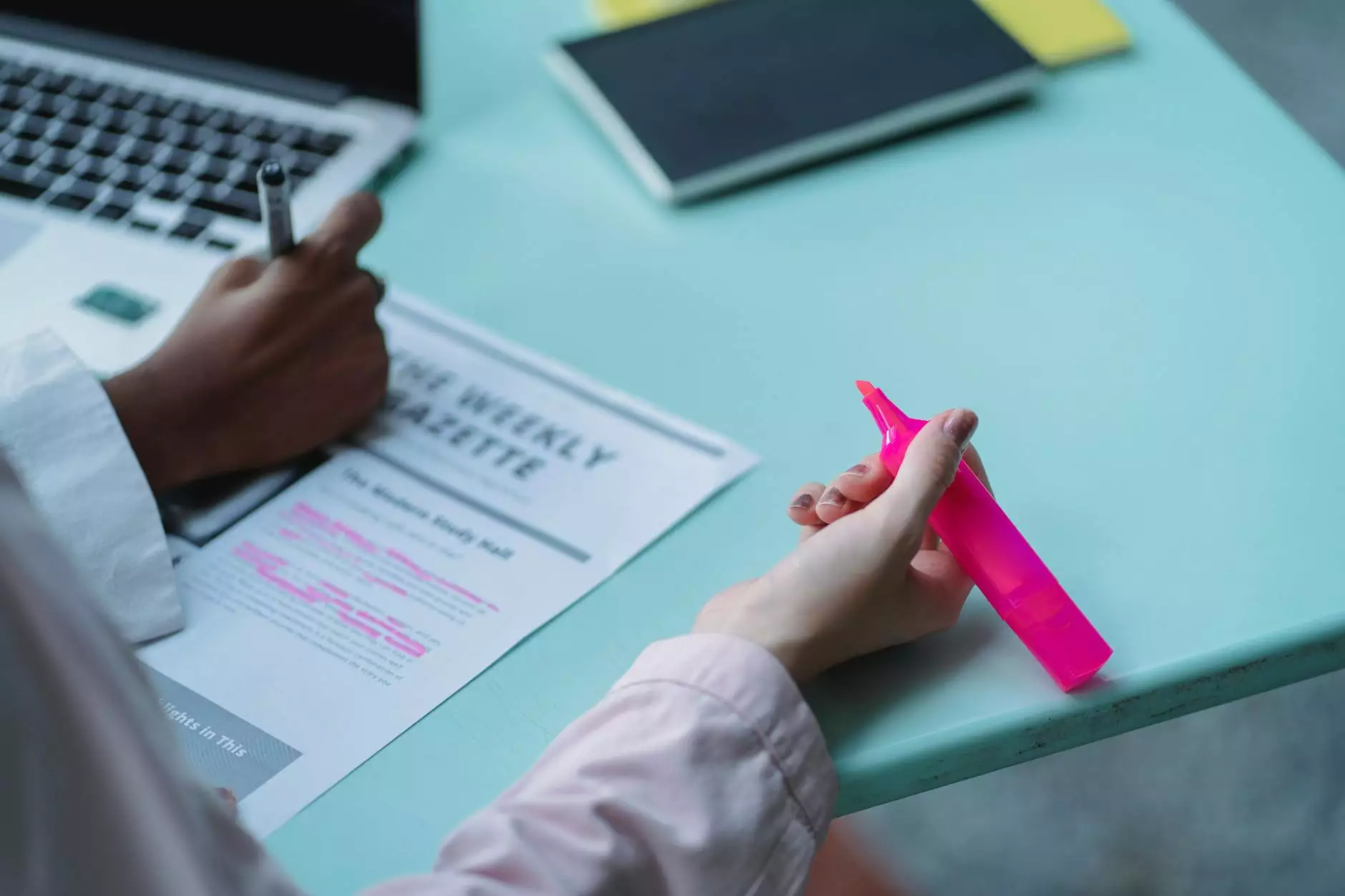
Introduction to Machine Learning Annotation
In the era of rapidly advancing technology, machine learning annotation has emerged as a crucial component in developing intelligent systems. At its core, machine learning annotation involves the process of labeling data to train machine learning models. This practice not only enhances the accuracy of algorithms but also plays a pivotal role in various domains, including home services, keys, and locksmith industries.
The Importance of Machine Learning in Business Operations
As businesses seek innovative ways to improve efficiency and customer satisfaction, machine learning techniques are becoming indispensable. By employing machine learning annotation, companies can transform vast amounts of raw data into insightful information, driving better decision-making processes.
Here are some key benefits of integrating machine learning into business operations:
- Improved Decision Making: Businesses can leverage data-driven insights to refine their strategies.
- Enhanced Customer Experience: Personalized services help in retaining customers and building loyalty.
- Increased Operational Efficiency: Automation of tasks leads to time and resource savings.
- Innovative Product Solutions: Data analysis helps in identifying market trends and customer needs.
What is Machine Learning Annotation?
Machine learning annotation is the process of labeling data sets to make them understandable for machine learning algorithms. The data can come in various forms such as images, text, or audio. By accurately annotating data, companies can train their models to recognize patterns and make predictions.
For instance, in the context of the locksmith industry, machine learning can be employed to analyze security patterns and predict potential security breaches. However, to achieve these results, the data that feeds into the system must be meticulously annotated.
The Process of Machine Learning Annotation
The annotation process involves several critical steps:
- Data Collection: Gather relevant data that needs to be annotated.
- Data Cleaning: Ensure that the data is free from errors and inconsistencies.
- Labeling: Apply appropriate labels to the data, which may involve human annotators or automated tools.
- Quality Assurance: Validate the annotations to maintain accuracy and consistency.
- Model Training: Use the annotated data to train machine learning models effectively.
Applications of Machine Learning Annotation in Home Services
In the home services industry, machine learning annotation can be particularly beneficial. Here’s how:
1. Predictive Maintenance
By analyzing data from home appliances, machine learning models can predict when maintenance is required, reducing the risk of unexpected failures.
2. Customer Behavior Analysis
Annotated data collected from customer interactions can provide insights into preferences and behaviors, enabling businesses to tailor their services.
3. Enhanced Security Solutions
In the locksmith sector specifically, machine learning models can analyze crime data to predict trends in theft, helping security service providers to better allocate resources and devise prevention strategies.
Challenges in Machine Learning Annotation
Despite its advantages, machine learning annotation comes with its own set of challenges:
- High Labor Costs: Manual data labeling can be time-consuming and expensive.
- Data Quality Issues: Poor quality data can lead to inaccurate models.
- Scalability: As data volumes grow, annotation processes need to scale accordingly.
- Subjectivity: Different annotators may interpret data differently, leading to inconsistencies.
Finding the Right Annotation Solutions
To successfully implement machine learning annotation, businesses must adopt the right strategies. Here are some tips to consider:
- Choose the Right Tools: Invest in reliable annotation tools that cater to your specific needs.
- Outsource When Necessary: Consider outsourcing to specialized companies if in-house resources are limited.
- Focus on Quality: Implement strict quality control measures to ensure data integrity.
- Train Your Team: Continuous training on the latest technologies and best practices is essential for maintaining an edge.
The Future of Machine Learning Annotation
The future landscape of machine learning annotation looks promising, with advancements in AI and automation set to revolutionize the process. Innovations such as semi-automated annotation tools are already reducing the burden on human annotators by accelerating the labeling process while preserving accuracy.
Furthermore, as businesses in various sectors, including home services and locksmithing, recognize the value of data, the demand for high-quality annotated data is expected to rise. This presents an opportunity for companies like Keymakr.com to leverage machine learning annotation for enhancing service delivery and operational efficiency.
Conclusion
In summary, machine learning annotation is more than just a technical necessity; it is a vital strategy for businesses looking to thrive in a data-driven world. By effectively annotating data, companies can harness the true potential of machine learning, ultimately enhancing customer experiences and driving growth.
Keymakr.com stands at the forefront of the home services and locksmith industry, poised to integrate machine learning and annotation processes to provide cutting-edge solutions. As the landscape evolves, embracing these innovations will pave the way for increased efficiency and customer satisfaction.