Unlocking the Potential of Image Data Annotation for Your Business
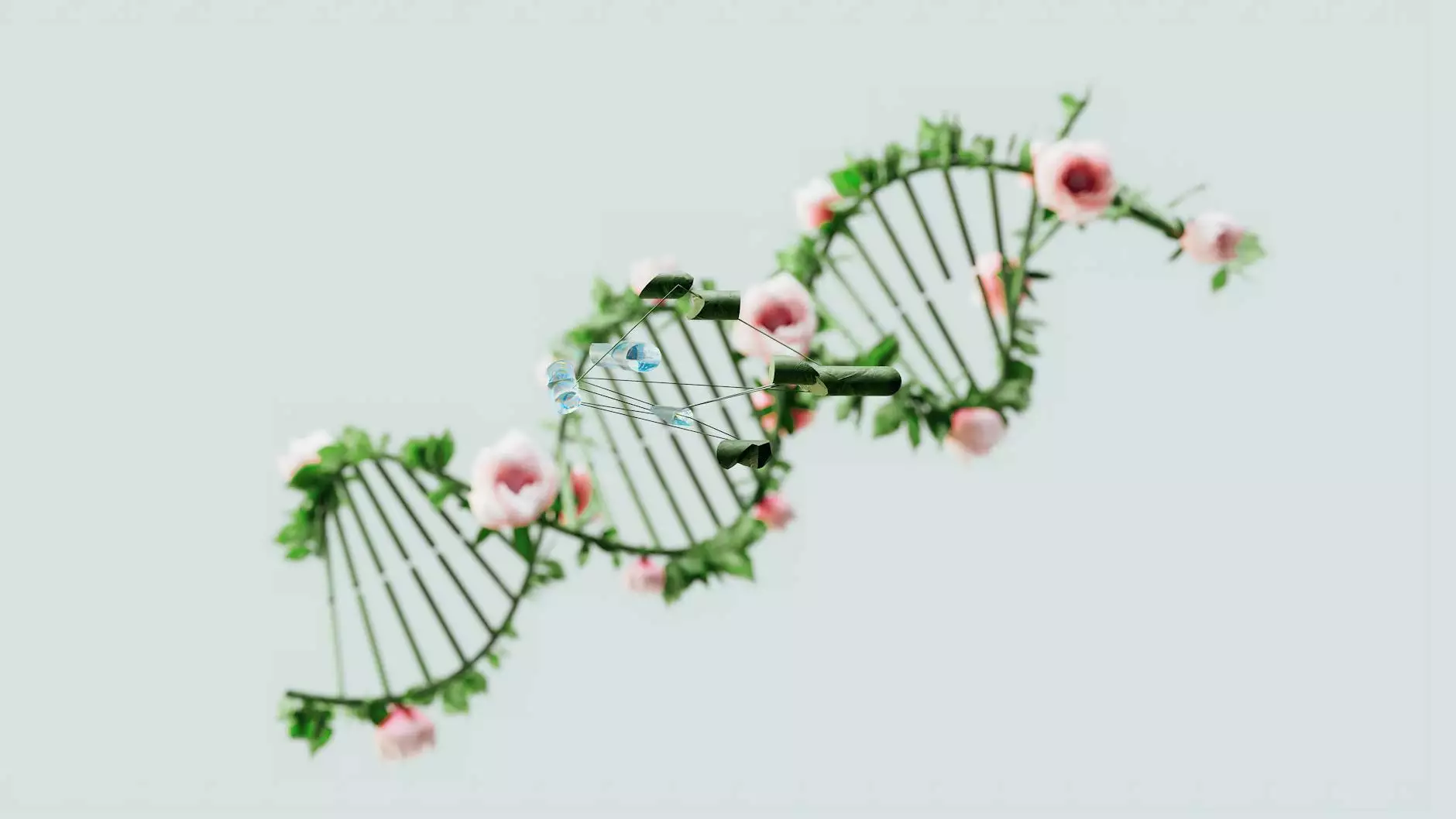
Image data annotation is a critical step in the development of artificial intelligence (AI) and machine learning (ML) models. As industries lean more into technological advancements, understanding the intricacies of data annotation can significantly improve the outcomes of AI initiatives. This guide serves to explore the significance of image data annotation and how tools like those provided by KeyLabs.ai can transform your approach to AI.
What is Image Data Annotation?
At its core, image data annotation involves labeling images to create a dataset that can be used to train machine learning algorithms. This process creates a bridge between raw data and its usability in teaching AI how to interpret and understand visual information. The pivotal role of image data annotation cannot be understated, as accurate annotations directly influence the performance and effectiveness of AI systems.
The Importance of Image Data Annotation in AI Development
As the AI landscape continues to evolve, the demand for high-quality annotated datasets has surged. Here are key reasons why image data annotation is essential:
- Enhanced Accuracy: Well-annotated images lead to better model accuracy. AI systems need precise labels to make correct predictions.
- Better Generalization: Annotations help models generalize from training data, allowing them to perform well on unseen data.
- Scalability: With the right annotation, businesses can scale their AI solutions and adapt to new images or video data quickly.
- Task Specialization: Different AI applications require specific types of annotations, such as object detection, segmentation, or classification.
Types of Image Data Annotation
There are various types of image data annotation techniques, each serving unique purposes depending on the AI model's needs:
1. Image Classification
In image classification, images are categorized based on labels. For instance, determining whether an image contains a cat or a dog falls under this category.
2. Object Detection
This technique identifies and localizes objects within an image, often providing bounding boxes around them. For example, detecting cars in an image of a street.
3. Image Segmentation
Image segmentation involves partitioning an image into multiple segments to simplify analysis, crucial for tasks where precise localization is necessary, like in medical imaging.
4. Landmark Annotation
Used particularly in facial recognition and human pose estimation, landmark annotation marks key points on images, aiding in analyzing features.
How KeyLabs.ai Enhances Your Image Data Annotation Process
KeyLabs.ai offers a comprehensive data annotation tool and platform that simplifies the image data annotation process. Here are some features of our platform:
- User-Friendly Interface: Our intuitive design allows users to perform annotations quickly and efficiently.
- Robust Quality Control: With built-in checking protocols, you can ensure high-quality annotations that boost your AI effectiveness.
- Flexible Integration: The platform easily integrates with existing workflows and supports various data formats.
- Scalability: Regardless of your project size, KeyLabs.ai can adapt to meet your annotation needs.
Best Practices for Effective Image Data Annotation
To achieve optimal results from image data annotation, consider the following best practices:
1. Define Clear Guidelines
Establishing clear rules for how images should be annotated helps maintain consistency across numerous datasets.
2. Utilize Automation
While manual annotation is necessary, leveraging automation can significantly speed up the process without compromising quality.
3. Select Experienced Annotators
Your annotation workforce should understand the required output and the context of the data they are working with.
4. Conduct Iterative Feedback Sessions
Feedback loops can help identify common errors and improve not just individual performance but the overall quality of annotations.
Challenges in Image Data Annotation
Despite its advantages, image data annotation is not devoid of challenges. Some common hurdles include:
- High Labor Costs: Manual annotations can be resource-intensive and expensive.
- Quality Assurance: Ensuring consistent quality across large datasets remains a significant challenge.
- Specialization Needs: Certain tasks may require specialized knowledge which can be hard to find within a general workforce.
The Future of Image Data Annotation
As AI systems become ever more sophisticated, the future of image data annotation looks promising but complex. Here are anticipated trends:
- Increased Use of AI in Annotation: Automation tools will become more prevalent in reducing manual effort involved in image data annotation.
- More Collaborative Platforms: Cloud-based solutions will facilitate team collaboration and real-time editing in annotation tasks.
- Growing Demand for Annotations: With the rise in AI applications, the need for various types of annotated datasets will soar.
Conclusion: Driving Your Business Forward with Image Data Annotation
The role of image data annotation in the AI ecosystem cannot be overstated. As businesses increasingly adopt AI technologies, understanding and implementing effective image annotation strategies will be vital. Utilizing robust platforms like KeyLabs.ai can streamline your processes, ensuring you have high-quality data to drive your AI initiatives forward. Embrace the power of image data annotation and position your business at the forefront of AI innovation.